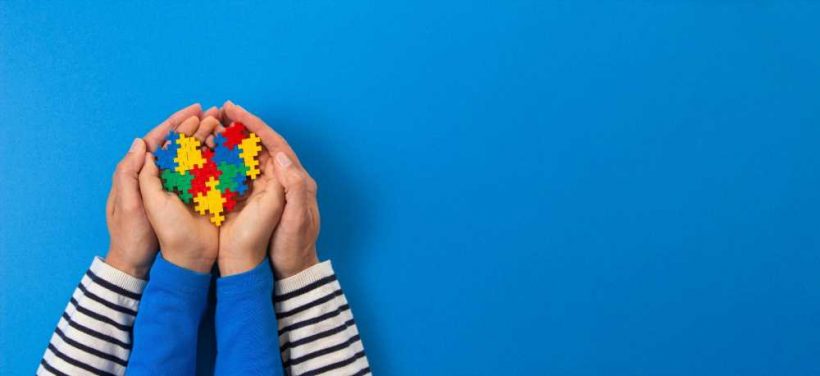
Early autism diagnosis breakthrough: machine learning uses eye movements on real and artificial faces
In a recent study published in Frontiers in Neuroscience, researchers developed an innovative autism spectrum disorder (ASD) detection technique for children by examining eye movement information for cartoon choices with machine learning.
Study: Machine learning-based early diagnosis of autism according to eye movements of real and artificial faces scanning. Image Credit: Veja/Shutterstock.com
Background
Early detection and treatment are necessary for ASD, a social condition marked by communication problems and compulsive behaviors.
In conjunction with machine learning algorithms, Eye-tracking has emerged as a critical tool for early screening and diagnosis.
Studies reveal that in ASD patients, gaze to stimuli with significant social value diminishes and rises, emphasizing the possibility of gaining an early and objective diagnosis if explored.
About the study
In the present study, given that aberrant gaze and developmental delay are early ASD indicators, researchers investigated whether minimal social stimuli such as cartoon activities may be effective for early ASD screening.
The researchers assessed ASD and typically developing (TD) children aged 12 to 60 months using eye-tracking tests based on films of cartoon characters and actual people. They analyzed the data from eye-tracking evaluations using a three-level hierarchical framework that included people, events, and interest areas.
Random forest (RF) classifiers were utilized for feature selection, and diagnostic data and flattened vectors were used as labels and features.
To analyze the impacts of the most important characteristics, logistic regression modeling was used. The Gesell development scale (GDS) was used to examine children's development.
Only individuals meeting the Autism Diagnostic Observation Schedule (ADOS) and the Diagnostic and Statistical Manual of Mental Disorders, Fifth Edition (DSM-V) criteria for ASD diagnosis and those with normal hearing and vision who could undertake eye movement evaluations were included in the analysis. Individuals with other psychiatric disorders and adverse physical health-related concerns were excluded from the study.
Eight soundless videos were used in the eye-tracking studies. The videos were divided into two sides by two rectangular sections, with one side featuring a cartoon figure performing motions such as nodding, dancing, scratching the neck, blowing a kiss, jumping, clapping hands, nodding while stretching thumbs, and skipping rope. On the other side, a real person impersonated the cartoon character.
To ensure that the attire and motions in the cartoon were equivalent to those among the actual humans, the cartoon and real human beings were simultaneously employed in the stimulus design. Fixation refers to a cluster of gaze locations near time (60 ms) and space. Gaze referred to the non-saccade movement status, whereas fixation referred to the non-saccade movement status.
Results
In total, 161 children (44 typically developmental children and 117 with ASD) were recruited, among whom the mean age was 40 months, and 47 were female. 70% and 30% of the data obtained were used for training and testing, respectively. ASD group children were significantly younger and showed substantially lower GDS scores than TD group children.
The overall values for recall, precision, and accuracy were 0.8, 0.7, and 0.7, respectively, with an area under the curve (AUC) value of 0.8. Attention to human-associated features connected favorably with ASD diagnosis, but cartoon fixation length correlated negatively with ASD diagnosis.
Head glance time and body revisited when cartoons clapped their hands, the cartoon sequence. At the same time, they scratched their necks, counted, glanced, and nodded while thumb stretching, and the total for people while dancing activities were negatively related to ASD.
People-initial-fixation-time while the individuals were dancing, cartoon sequences while the cartoons nodded, and cartoon character-fixation-duration while blowing kisses were the traits that indicated favorable connections with ASD. Furthermore, the greatest saccade velocity was associated with an ASD diagnosis.
ASD children loved cartoon faces far more than TD kids. A probable explanation for ASD children's love for cartoons is that they do not need social contact. The commonality of autism spectrum disorders in cartoon character processing might be linked to harm to social interaction abilities.
The findings revealed that the duration of people-first focus during dancing was strongly connected with the diagnosis of ASD. The explanation may be that actual individuals had a broader variety of motions, which youngsters were more drawn to.
Conclusions
Overall, the study findings showed that eye-tracking techniques and machine learning algorithms have shown promise in detecting ASD in young generations.
While actual human glancing behaviors like body revisits and head look length are associated with ASD, cartoon-related glancing behaviors like cartoon sequence and cartoon fixation times are not.
These actions are socially significant because children less interested in actual individuals or human parts are more likely to have ASD. Computer-aided approaches, such as cartoon characters, should be investigated further in early diagnosis and special education.
-
Meng F, Li F, Wu S, Yang T, Xiao Z, Zhang Y, Liu Z, Lu J, and Luo X (2023) Machine learning based early diagnosis of autism according to eye movements of real and artificial faces scanning. Front. Neurosci. 17:1170951. doi: 10.3389/fnins.2023.1170951. https://www.frontiersin.org/articles/10.3389/fnins.2023.1170951/full
Posted in: Child Health News | Device / Technology News | Medical Science News | Medical Research News | Medical Condition News
Tags: Autism, Children, Dancing, Diagnostic, Education, Eye, Hearing, Machine Learning, Neck, Neuroscience
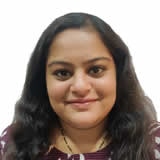
Written by
Pooja Toshniwal Paharia
Dr. based clinical-radiological diagnosis and management of oral lesions and conditions and associated maxillofacial disorders.